The Importance of Data Collection and Forecasting in the Energy Industry
Denali-ep
| 14 de octubre de 2024
The Importance of Data Collection and Forecasting in the Energy Industry
Introduction
In the energy industry, data collection and forecasting play a crucial role. These processes not only help optimize resource use but also enable companies to anticipate and adapt to changes in energy demand and supply. In this article, we will explore the significance of these processes, the methods used, and the benefits they offer.
What is Data Collection?
Definition and Relevance
Data collection is the process of gathering relevant information for a specific purpose. In the energy industry, this information may include historical consumption data, real-time data on energy generation and distribution, and future projections based on various variables. Accurate and timely data collection is essential for informed and strategic decision-making.
Types of Data in the Energy Industry
Historical Data
Historical data includes information about energy consumption and production over time. This data is crucial for identifying patterns and trends.
Real-Time Data
Real-time data is vital for operational management, enabling companies to monitor and adjust their operations on the go.
Projected Data
Projected data is based on predictive models and helps companies anticipate future market behavior.
Data Collection Methods
Sensors and IoT Devices
The Internet of Things (IoT) allows sensors to be installed on energy systems and equipment, providing accurate, real-time data.
Satellites and Drones
Satellites and drones offer a broad and detailed view of energy infrastructures, aiding in monitoring and analysis.
Human and Manual Sources
Despite technological advancements, human and manual sources remain important for gathering qualitative and contextual data.
Importance of Data Quality
Accuracy
Data accuracy is crucial to ensure that the analyses and decisions based on the data are reliable.
Integrity
Integrity refers to the completeness and correctness of data. Incomplete or inaccurate data can lead to poor decisions.
Consistency
Consistency ensures that data remains uniform and coherent over time and across different sources.
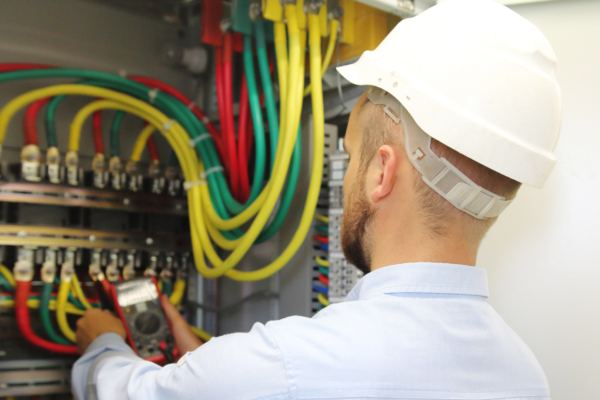
Forecasting in the Energy Industry
What is Forecasting?
Forecasting is an estimate or prediction about future events based on data and analysis. In the energy industry, forecasts can predict demand, production, and energy prices.
Types of Forecasts
- Short-Term Forecasts: Used for daily operations and immediate adjustments.
- Mid-Term Forecasts: Help with resource planning and maintenance.
- Long-Term Forecasts: Used for strategic planning and investments.
Tools and Technologies Used
Data Analysis Software
Tools like Tableau and Power BI allow companies to visualize and analyze large volumes of data.
Predictive Models and Machine Learning
Machine learning helps create more accurate predictive models by learning from historical data and adjusting to new information.
Artificial Intelligence
Artificial intelligence (AI) can process large amounts of data and provide detailed and fast insights.
Benefits of Forecasting
Resource Optimization
Forecasting allows companies to use their resources more efficiently, reducing waste and improving productivity.
Cost Reduction
Accurate forecasts help avoid unnecessary costs by optimizing energy and resource use.
Improved Decision-Making
Reliable data and forecasts enable leaders to make informed and strategic decisions, enhancing the company's competitiveness.
Use Cases in the Energy Industry
Renewable Energy
Forecasts help manage the intermittency of renewable sources like solar and wind, improving their integration into the grid.
Grid Management
Data collection and forecasting enable more efficient and reliable grid management.
Demand and Supply Prediction
Forecasting allows companies to anticipate changes in demand and adjust the energy supply accordingly.
Challenges in Data Collection and Forecasting
Data Security
Protecting collected data is crucial to avoid security breaches and maintain consumer trust.
Model Complexity
Predictive models can be complex and require constant updates to maintain accuracy.
Data Variability
Data can vary significantly, which poses a challenge for creating accurate and reliable models.
Regulations and Compliance
International Regulations
Companies must comply with international regulations that ensure transparency and responsibility in data management.
Compliance Requirements
Meeting compliance requirements is essential to avoid penalties and maintain operational efficiency.
The Future of Data Collection and Forecasting
Technological Innovations
Technological innovations continue to transform how data is collected and analyzed in the energy industry.
Emerging Trends
Trends such as the use of big data, AI, and machine learning are redefining the capabilities of forecasting and analysis.
Conclusion
Data collection and forecasting are fundamental to the success and sustainability of the energy industry. These processes enable more efficient management, reduce costs, and improve strategic decision-making. With technological advancements, companies have the opportunity to continuously enhance their analytical and forecasting capabilities, ensuring a more reliable and sustainable energy future.
Why is data collection important in the energy industry?
Data collection is essential for making informed decisions about energy production, distribution, and consumption. It helps companies track trends, optimize resource use, and improve operational efficiency by providing accurate, real-time insights into energy systems.
What are the common methods for data collection in the energy industry?
Data can be collected using various methods, including IoT sensors, satellites, drones, and manual reports. IoT sensors provide real-time data, while satellites and drones offer a broader view of energy infrastructure. Manual data collection is often used for qualitative insights.
How does forecasting benefit energy companies?
Forecasting helps energy companies anticipate future demand, optimize resource allocation, reduce operational costs, and make strategic investment decisions. It also improves the integration of renewable energy by predicting fluctuations in supply and demand.
What technologies are used for energy forecasting?
Energy forecasting relies on technologies such as machine learning, artificial intelligence (AI), and data analysis software like Tableau and Power BI. These tools help process large amounts of data, create predictive models, and provide actionable insights.
What challenges do companies face in data collection and forecasting?
Common challenges include ensuring data accuracy, maintaining data security, managing the complexity of predictive models, and dealing with the variability of energy data. Additionally, staying compliant with industry regulations is critical to avoid penalties.